
The structure, physical and chemical properties x of these molecules are known. Search for common propertiesĪ certain class of molecules has a positive medical e ect. Usually a simple interpolation will solve this problem.įor more complicated relations, approximations with polynomials, higher spline functions or orthogonal functions are useful.Įxample 136. In the application we want to find for a given input R an estimate of T. In the teaching process which here is called calibration, a sample of corresponding values R i, T i is acquired. The resistance R of a wire is used for a measurement of the temperature T. We can not learn much from erratic distributions. In our approach this translates into the requirement that the responses are similar for input vectors which are close. Learning which exceeds simple memorizing relies on the existence of more or less simple relations between input and response: Similar input corresponds to similar response. The region of interest should be covered with input vectors homogeneously, and we should be aware that the accuracy of the estimate decreases at the boundary of this region. Consequently, the training sample should be as large as possible or a ordable. When we ask for the response to an arbitrary continuous input x, usually itsĮstimate yˆ(x) will be more accurate when the distance to the nearest input vector of the training sample is small than when it is far away.
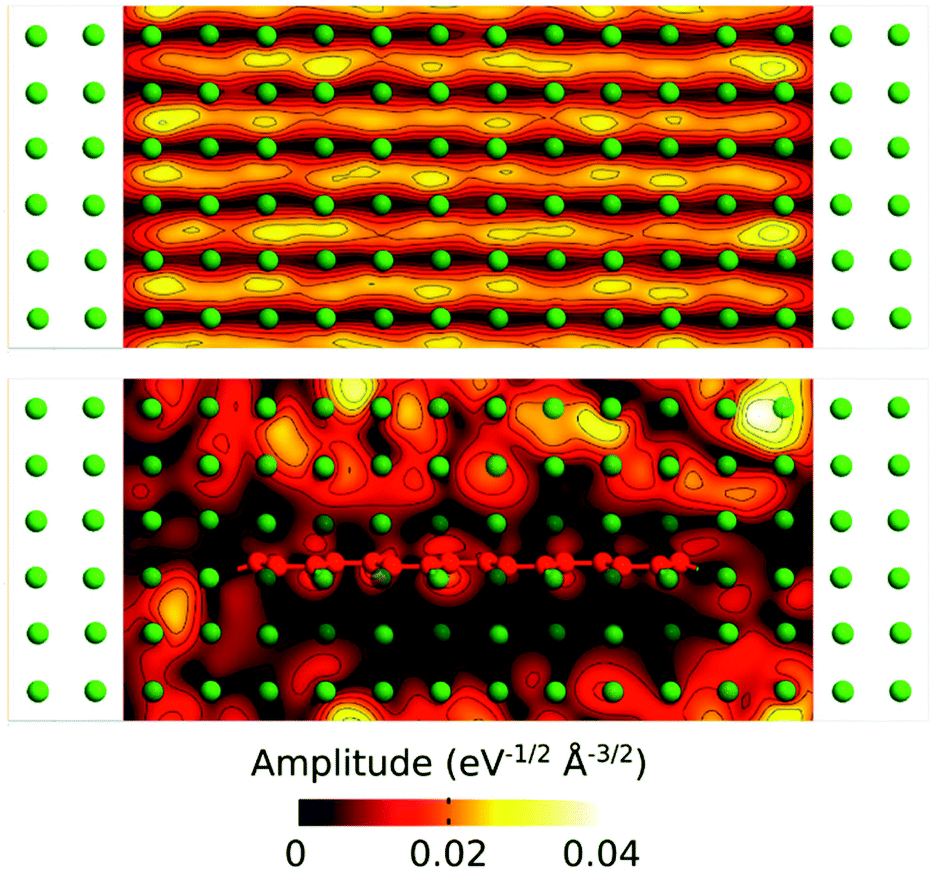
, where for each input vector x i the response y i is known. The teaching process, called training, uses a training sample Like a human being, a computer program learns from past experience. The output can also contain several components or consists of a single real or discrete ( Yes or No) variable. Normally, it is a metric (quantifiable) quantity but it could also be a categorical quantity like a color or a particle type. Usually, each input consists of several components ( attributes, properties), and is written therefore in boldface letters. The input is also called predictor, the output response.
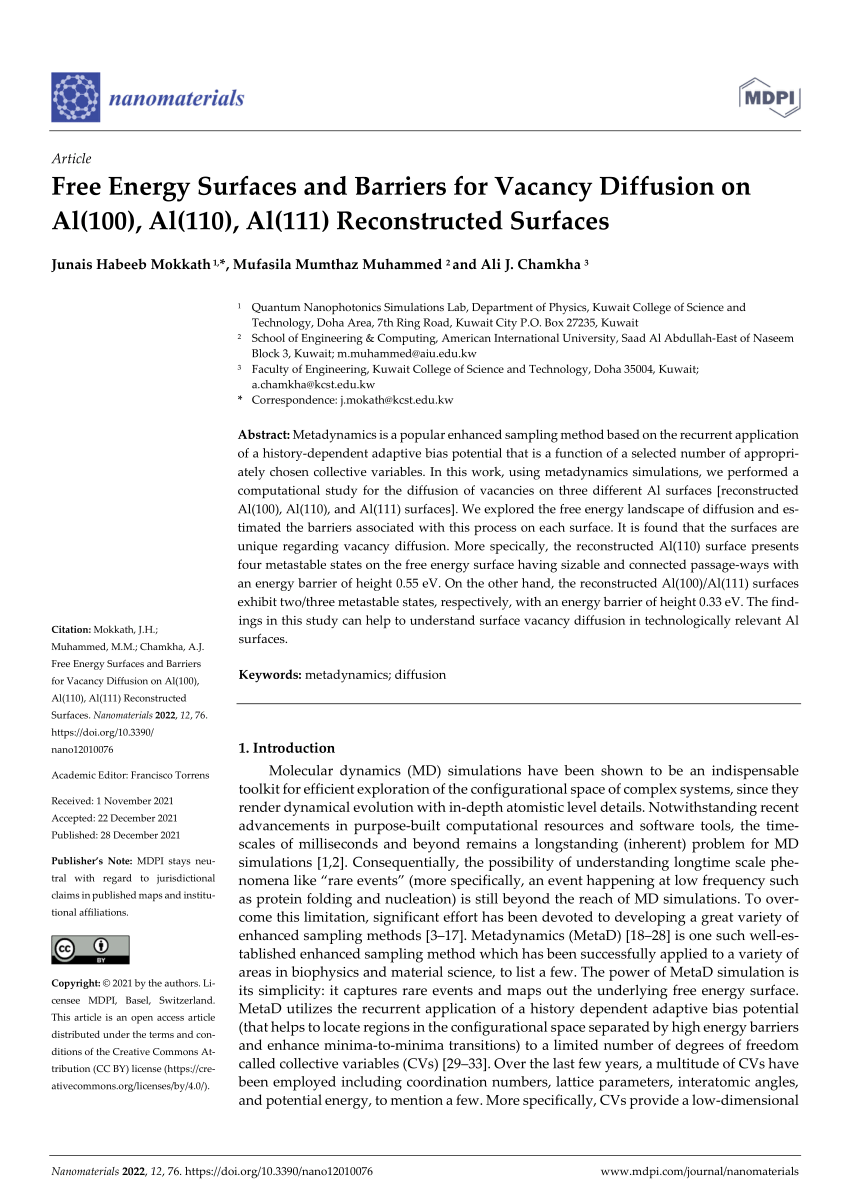
Let us now specify the type of problems which we discuss in this chapter: For an input vector x we want to find an output yˆ. The techniques and programs that allow computers to learn and to classify are summarized in the literature under the term machine learning. The achievements of the so-called artificial intelligence are still rather moderate in most areas, however a substantial progress has been achieved in the fields of supervised learning and classification and there computers profit from their ability to handle a large amount of data in a short time and to provide precise quantitative solutions to well defined specific questions. If this is not the case, we have to rely on approximations, like interor extrapolations.Īlso computers, when appropriately programmed, can perform learning processes in a similar way, though to a rather modest degree.
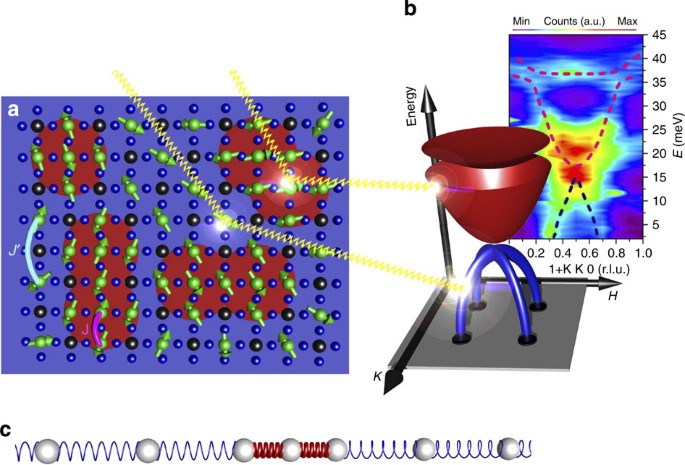
This is relatively easy, when the laws of nature governing a specific relation have been discovered. But the process of learning – of children and adults – is not restricted to the development of the ability merely to classify but it includes also the realization of relations between similar objects, which leads to ordering and quantifying physical quantities, like size, time, temperature, etc. This process partially proceeds through explanations by parents and teachers (supervised learning), but partially also by cognition of the similarities of di erent objects (unsupervised learning). In the process of its mental evolution a child learns to classify objects, persons, animals, and plants.
